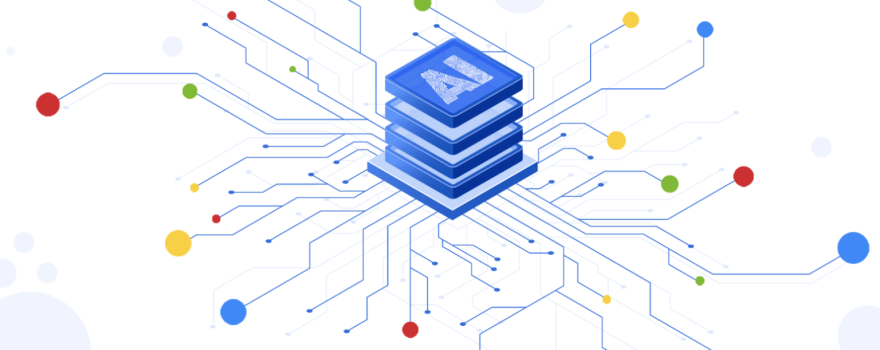
The PaLI model, pre-trained on WebLI, is said to deliver the highest quality image and language tests such as COCO-Captions, CC3M, TextCaps, nocaps, VQAv2 and OK-VQA.
In a blog post last week, Google AI introduced ‘PaLI,’ a collaboratively scalable multilingual language-image model that is trained to perform various tasks in more than 100 languages.
The goal of the project is to explore how language and vision models interact at scale, with a particular focus on the scalability of language and image models.
The newly introduced model will perform tasks spanning vision, language, multimodal image, and language applications such as visual question answering, object identification, image captioning, OPC, and textual reasoning.
The researchers used a collection of publicly available images that includes automatically collected annotations in 109 languages, called the ‘WebLI’ dataset. The PaLI model, pre-trained on WebLI, is claimed to provide stateCurrent performance on complex image and language tests such as COCO-Signatures, SSZM, TextCaps, nocaps, VQAv2 and OK-VQA.
The architecture of the PaLI model is considered simple, scalable, and reusable. Input text is processed using a Transformer encoder along with a Transformer autoregressive decoder, which generates the output text. The Transformer encoder input additionally includes “visual words” that represent the image processed by the Vision Transformer (ViT).
Scaling deep learning research shows that larger models need more datasets to train effectively. According to the blog, the team created ShoeBLI-a multilingual image dataset made from images and text readily available on the public Internet-to unlock the potential of interpreting language images.
It goes on to add that “WebLI scales text language from English-language datasets to 109 languages, allowing us to perform tasks in many languages. The data collection process is similar to that used in other datasets, such as ALIGN and LiT, and allows us to scale the WebLI dataset to 10 billion images and 12 billion alt-texts.”
PaLI is said to outperform multilingual visual captioning of previous models and visual question answers. The team hopes this work will spur further research into multimodal and multilingual models. The researchers believe that vision and language tasks require large-scale models in multiple languages. In addition, they argue that further scaling of such models is likely to contribute to these tasks.